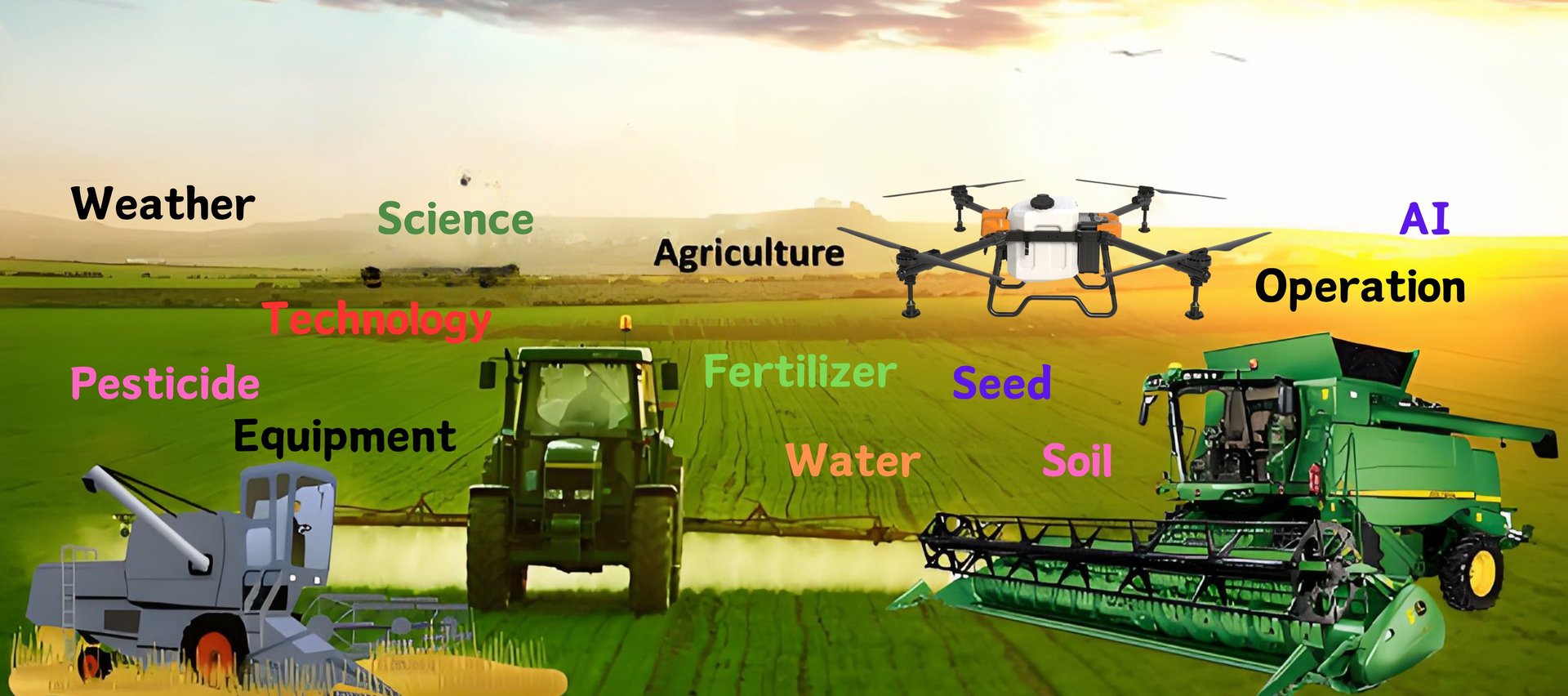
The Application of Big Data in Agricultural Production
In recent years, the agricultural sector has undergone a profound transformation fueled by technological advancements, among which big data stands out as a game-changer. The advent of big data analytics in agriculture has opened avenues for more efficient production methods, better resource management, and enhanced decision-making processes. This essay explores the various applications of big data in agricultural production, highlighting its significance in addressing the challenges faced by farmers today.
Precision Agriculture
One of the most impactful applications of big data in agriculture is precision farming. This approach relies on data collection from multiple sources, such as satellite imagery, GPS technology, and sensor networks. By analyzing this data, farmers can gain insights into soil health, crop conditions, weather patterns, and pest infestations. For example, using satellite imagery, farmers can precisely monitor crop growth at various stages, allowing them to apply fertilizers and pesticides more efficiently. Instead of blanket spraying fields, targeted applications can be made based on data-driven decisions, reducing waste and minimizing environmental impact.
Yield Prediction and Crop Management
Big data analytics significantly enhances yield predictions, allowing farmers to make informed decisions about planting and harvesting. Historical data combined with real-time analytics can forecast crop yields with remarkable accuracy. For instance, companies like IBM and Climate Corp utilize algorithms that analyze weather conditions, soil moisture levels, and historical crop performance to provide farmers with actionable insights. This ability to predict yields enables better inventory management, reduces food waste, and helps farmers make informed choices about market timing.
Resource Optimization
Water scarcity is a pressing issue in agriculture, making efficient water management crucial. Big data can help optimize irrigation practices through data-driven insights. Smart irrigation systems equipped with sensors collect data on soil moisture and weather conditions, allowing for precise irrigation scheduling. This not only conserves water but also ensures that crops receive the right amount of moisture at critical growth stages. By reducing water usage, farmers can improve sustainability and lower costs, contributing to a more resilient agricultural system.
Pest and Disease Management
Pests and diseases pose significant threats to crop production. Big data plays a crucial role in early detection and management of these threats. By analyzing historical data on pest outbreaks, weather patterns, and crop health, farmers can anticipate potential infestations. For instance, predictive analytics can identify conditions that are conducive to pest proliferation, allowing farmers to take preventive measures before an outbreak occurs. This proactive approach reduces the need for chemical treatments and helps maintain healthier ecosystems.
Supply Chain Optimization
Big data also extends beyond the farm gate, impacting the entire agricultural supply chain. By analyzing data from various stakeholders, including suppliers, distributors, and retailers, farmers can optimize their logistics and distribution strategies. For example, big data can help identify the best markets for selling produce based on demand forecasts and price trends. Improved supply chain visibility allows farmers to reduce losses due to spoilage, ensuring that fresh produce reaches consumers in optimal condition.
Economic Viability and Risk Management
In an industry heavily impacted by climate change and market fluctuations, big data provides tools for economic viability and risk management. By utilizing data analytics, farmers can assess potential risks, such as droughts or market downturns, and develop contingency plans. For instance, farmers can access models that simulate various scenarios, helping them choose the best course of action under uncertain conditions. This strategic approach not only secures farmers’ livelihoods but also promotes resilience within the agricultural sector.
Conclusion
The application of big data in agricultural production represents a paradigm shift in how farming is approached. By harnessing the power of data analytics, farmers can make informed decisions that enhance productivity, sustainability, and profitability. As the global population continues to grow and the demand for food escalates, the integration of big data into agriculture will be essential for meeting these challenges. Embracing this technological revolution will empower farmers to optimize their operations, manage resources more effectively, and contribute to a more sustainable food system for future generations.